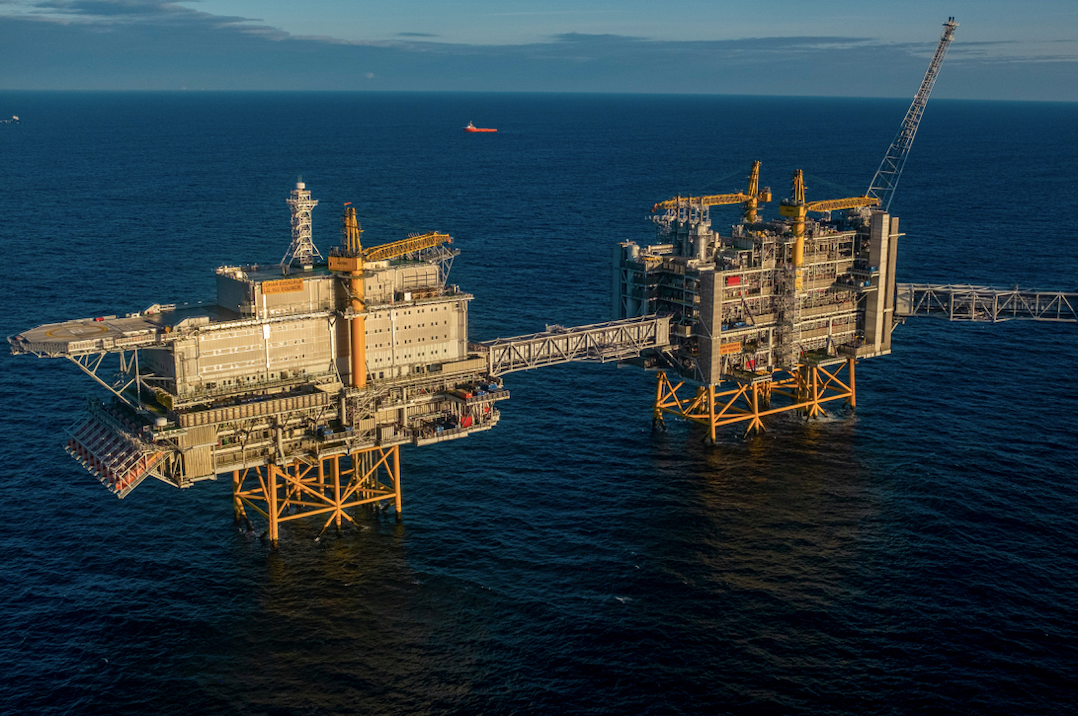
Automation in the offshore oil and gas industry has been on a rise with sensor-based technologies at the front line of advancements. In precarious environments, AI has replaced humans to increase worker safety. Robots have been employed to check for rig leaks and sensors can now prompt the predictive maintenance of machinery.
Reliance on automation also means feeding multitudes of data to processing systems for smooth functioning of offshore facilities. On one hand, AI analysis has aided the human interpretation of data to find low-grade seismic faults for oil extraction, otherwise invisible to the human eye. On the other, there are questions about sanctity of data, margins of error, and possible uncertainties that AI is not trained for.
What happens when AI receives faulty data from sensors or develops a bias? How does AI respond to situations of uncertainty? Founder of Indian AI technologists Matra, Ajay Davessar, unravels the challenges of working with AI and the correctional technologies that the offshore industry hopes will change the game.
Ashima Sharma: A lot of data generated from IoT sensors is now used in predictive maintenance of machinery and also assessment of future oil assets. What was the oil exploration landscape before the use of artificial intelligence (AI)?
Ajay Davessar: In the first stage of oil and gas exploration, a geology prediction determines the nature of the rock in the ocean bed. This prediction is based on the data that geologists and other scientific experts gather from IoT devices and sensors. Currently, a lot of systematic data is captured to determine measures such as, how porous is the rock? Is it a sandstone or a shale rock? All these inputs let the experts examine the chances of finding oil and in what quantity. Before AI, this was all being done by linear algebra, statistics or probabilistic equations.
How well do you really know your competitors?
Access the most comprehensive Company Profiles on the market, powered by GlobalData. Save hours of research. Gain competitive edge.
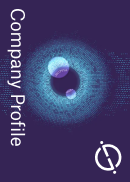
Thank you!
Your download email will arrive shortly
Not ready to buy yet? Download a free sample
We are confident about the unique quality of our Company Profiles. However, we want you to make the most beneficial decision for your business, so we offer a free sample that you can download by submitting the below form
By GlobalDataThe main challenge that AI undertakes occurs in a phase called object identification, labelling masses beneath the earth’s crust. Earlier, object identification was done by a lot of statistical techniques, but then AI and machine learning were introduced. Now object identification is being done by a specific technique in AI called computational neural networks, or CNNs. These in particular are very useful for pattern and object identification.
See Also:
For example, you take an x-ray of the earth’s crust and also get some images from under the surface. Now the objects in the image are identified by certain patterns. If the density of the dots, or the grey area in a specific part of the x-ray is more, you identify it as a rock and not fluid, just like in x-rays or CT scans of the body. Similarly, CNNs help in identifying the nature of object or whether it is an outside body/intrusion.
Earlier, it was a Herculean task to determine the nature of the object because of the magnitude of data components that go into it. Many times, the data you have is so weak that these patterns don’t emerge or are not visible to the human eye. Detection of faults or intrusions in the earth’s surface thus becomes difficult if one is to rely on humans to catch these minuscule details.
Ashima Sharma: How has AI aided predictive modelling for the offshore industry?
Ajay Davessar: The most significant way it has aided the offshore industry is seen in reservoir modelling. In this scenario, we are uncertain about how the reservoir will behave once we drill it. But AI techniques like decision trees, graphic processing units and support vector machines are helping to predict outcomes.
Add on to it, the data from the logs of oil wells. Let’s say we make a model as an oil and gas company that predicts how a reservoir will behave. But when it starts operating, the logs tell a different story.
This means your model was accurate maybe only up to 70% or 80%. With machine learning, the feedback loops have now started emerging in the oil and gas industry. Large numbers of people are now benefiting from capturing real production data. The data learning loops are now making our models more and more accurate because machine learning, as the name says, is all about learning continuously.
Ashima Sharma: There is a lot of new research around the use of attention mechanisms to find low-grade faults in the offshore explorations. Could you elaborate on the technique?
Ajay Davessar: Attention mechanism is a technique where you add weights to the signals you receive to amplify the useful output.
Attention mechanism has three components to it. One is query, second is the key, and third is the value. Let’s say your query is to find out the nature of the rock. The key is the kind of data related to the query that will answer it, for instance telling me whether the rock is shale or porous. The value is the weight you attach to a certain attribute in order to better determine the validity of your find.
Attention mechanism use is growing in the oil and gas industry because it not only detects faults, but also finds anomalies. There could be various anomalies in the readings of the sensors or the equipment’s performance. Before failure, equipment is likely to give you a very weak indication of damage. If you’re putting an equipment, miles down into the earth, you want to be sure about any anomaly or preventive maintenance you need to do.
Ashima Sharma: Machine learning and AI and are based on learning from and responding to patterns in huge amounts of data. How does AI deal with situations of uncertainty, especially in an industry like oil and gas?
Ajay Davessar: When you send something out there in the field, you can’t model everything that your machines could encounter. Similarly, there is a technique in AI called Monte Carlo, which predicts situations that are complex and where the outcome is very uncertain. It is named after the Monte Carlo casino in Monaco, which is known for its games that have very random outcomes.
In Monte Carlo simulations, random sampling is used. In oil and gas, AI uses Monte Carlo techniques to generate situations vary rapidly, which you can’t create otherwise through data. So, it can throw up situations which you did not include in your hypothesis and helps you prepare better offshore exploration models.
Based on the spatial data for your assets, that includes the oil rig, the machinery, the supply chain etcetera, it will try and create scenarios that are combinations of a multitude of factors as to what can go wrong in what combination.
Ashima Sharma: Have you encountered any cases of faulty data? How can this affect producers, and how can it be corrected?
Ajay Davessar: It is a huge risk factor to both humans and environment if the machine learning interface encounters errors in data.
Companies have been treading very cautiously with AI, simply because there are too many processes at play. For instance, we are monitoring the flow of fluid into pipes that transport oil, checking consumption, and assessing how gas emissions are behaving, all at the same time. We have encountered situations where offshore predictive models have not worked at all.
Industry experts are now creating disaster recovery plans. Let’s say one operation shuts down; but you have a disaster recovery plan, which is a hot standby. This means the system almost instantaneously takes over without even realizing that the new system has taken over. The cold standby is where you have to manually tweak some algorithms to get it to work.
The latest technology here is the zero-user interface (zero UI). Let us say you are stuck in a place and there is fire around and you. You don’t know what to do, which lever to pull, or which button to press. The zero UI will take over the systems. It will ask which part of the infrastructure you are in, for instance asking the kind of signs you are close to. It’s talking to you, and it’s observing.
Google Maps is a very small example of zero UI. You’re driving and the map is not relying on you to input your location. It simply says, take a left in a hundred meters. These are examples of zero UI, and this AI is largely being used as preparation for crisis management in the offshore industry.